The SaaS Metrics Blueprint: How to Define, Measure and Display What Actually Matters
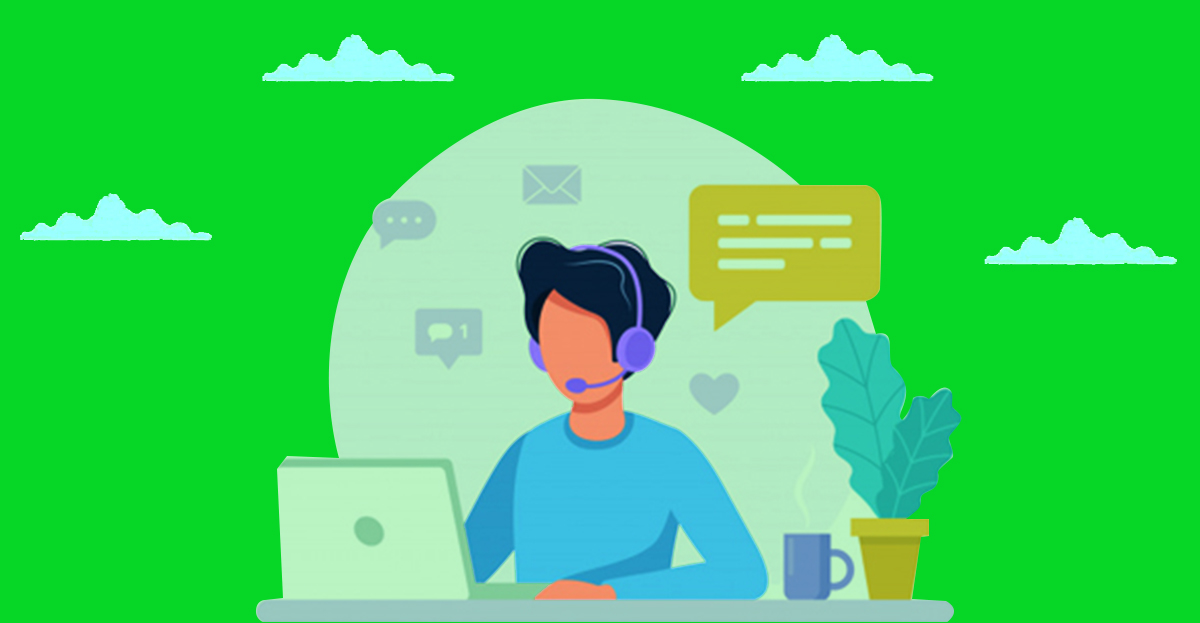
This is part in which we analyze and provide practical advice for SaaS sales organizations. In this blueprint, we’ll breakdown how to structure your SaaS metrics, and measure the right data for your business. Many organizations are excited about the amount of data that is flowing into their platform. However, with the explosion of data, they soon are lost on how to interpret it. This results in no action, or actions with adverse impact.
CASE IN POINT: Giving a car driver a fuel efficiency indicator (MPG) vs. a speedometer changes the driving behavior. This indicates that the way you manage a business is influenced by what you measure and display.
In this Blueprint, we’ll share the essential principles that ensure you measure data correctly and display the right data to manage your business.
STEP 1: Standardize on Where We Are Going to Measure
It is key to standardize where we are going to measure progress. Below is an overview of key SaaS metrics.
STEP 2: Standardize on Terminology
SUSPECT: A person who may be interested.
PROSPECT: A person who expresses interest by visiting a web-site for example.
MQL (Marketing Qualified Lead) A person who expresses interest and fits the profile.
MQA (Marketing Qualified Account) A company whom you have identified as benefiting from your service.
SQL (Sales Qualified Lead) A person who wants to take action and positively impact the situation.
SAL (Sales Accepted Lead) Verified by a sales pro that an impact can be achieved.
COMMIT: Mutual commitment to deploy a solution that will impact the problem.
LIVE: Client has been on boarded, on-time, within budget and solution is ready to deliver impact (plug-in installed, platform integrated). Sometimes first impact is delivered.
USE: Solution delivers impact again and again.
+/x/=: Customer is happy buys more of your service through renewal, upsell and cross sell.
STEP 3: Standardize on Definitions
Next step is to standardize on what you are measuring at this point. Below an overview.
STEP 4: Standardize on Approach; Inbound, Outbound, or Target
What are we going to do with this data? Below is an example of how to standardize your approach.
Inbound: Reactionary to an inbound request (MQL) from a customer who experiences a pain, such as a demo request, trial sign-up, pricing request. The client is often interested to resolve it asap making this very time sensitive requiring a response in <5 minutes.
The general response is Thank you for reaching out. How can I help you?
Outbound: Pro-actively identify a prospect who may experience a pain, establish a conversation to diagnose the problem.
The general response is I noticed that you are … may I ask are you experiencing this pain?
Common mistake: Mis-categorization of nurtured leads as MQLs thus causing the wrong action.
CASE IN POINT: A client downloads a white paper and is asked for the email to receive it. The organizations categorize this as an inbound lead and follow it up by an MDR who calls up <5 mins and asks “how can I help you?”. The client is caught off-guard and did not even read the paper. They are not want to talk to anyone yet and feels intimidated by the aggressive follow-up.
Instead, organizations should direct such a non-time sensitive development towards the outbound sales process. In this case, an SDR uses the download/nurture history as they reach out a few days later following research identifying relevance, present a use-case and an offer to help.
Target/ABM: Pro-active based on a pattern of wins and/or strategic nature of deals a list of accounts can be pursued (Marketing Qualified Accounts). Difference vs. Outbound is that you target multiple people in an account with an orchestrated approach involving multiple people from your own organization. This focused approach provides a better chance to stand-out.
Keys to success are:
- Pick the right account
- Research
- Orchestrate
- Executive involvement
Common mistake: Pick 1,000 accounts and target 3 people in each account (this is an outbound process).
STEP 5: Identify Volume Metrics
Common volume metrics:
- Prospecting metrics
- Web visitors
- Webinar attendees
- White paper D/L
- # MQL
- Demo requests
- Quote requests
- # SQL
- Meetings set
- Demo’s in progress
- Commits
- Deals/Seats (#)
- Consumption (%)
- MRR ($)
STEP 6: Identify Conversion Metrics (direct relationship)
Common conversion metrics:
Marketing Conversion rate: indicates health of a database. If conversion rates are low, marketing is likely spamming them.
LeadGen Conversion rate: indicates the priority of the pain a client is experiencing. If low, the pain is not a priority at this time.
Sales Acceptance rate: indicates how well your team is qualifying the deals. If too low means either bad quality or sales team is too selective in accepting.
Win rate: Indicates the quality of the sales team and/or the quality of the deals. If too low (1:10) bad deals/bad sales performance, if too nigh (1:2) not enough deals accepted.
STEP 7: Performance Metrics
Performance metrics are more complicated to obtain as they require strict obedience to metric definitions and an understanding of relationship. However, they are more indicative of the right behavior. A few practical examples are:
Pipeline generation: What campaigns generate the most pipeline? If we know which campaign generate the most pipeline we can invest more into these campaigns. For example, it is a common experience that smaller regional events generate less awareness nationwide but more qualified leads in the forecast.
Success Rate: Looking beyond the close
Using volume metrics, we would measure the number of deals/or revenue generated per salesperson – this is indicative of their effectiveness in winning deals.
Using conversion metrics we’d measure how many deals it would take to win a deal (win rate) – this is indicative of the efficiency of a salesperson.
However, these two metrics may still not be an indication of success; certain salespeople motivated by a growth-minded comp plan will go for the “quick close”. This is particularly present in high growth company who have a “win at all cost” attitude. What is not accounted for is the cost of winning a bad deal which is proving to be higher than expected.
Using performance metrics such as success rate provides an indication if the salesperson sold the right customer the right solution. This is indicative of the salesperson performing a proper diagnose, and recommending the right solution. It is known that these deals spend more time in the education stage vs. being rushed through the pipeline.
Onboarding Impact: Most SaaS businesses only make a profit on a client many months into the deal. As many as 1-2 years even. If a great onboarding experience is lacking a client will churn resulting in the highest losses. Companies that pursue profitability have to look at two key moments that create an incredible impact on the opportunity:
Time to first value: The time it takes from Commit to LIVE, and how was that time spent?
Orchestration: A quality experience is where we re-educate the customer and set expectations of how the next 6-12 months will look like.
CASE IN POINT: How to use data (Problem vs. Solution)
- Problem: Measuring of related sales metrics against different points (SAL and SQL)
- Solution: Visualize what you are measuring – must measure against the same (SQL)
- Problem: See an increase in logo churn <90 days after close
- Solution: Check your sales cycle – if it’s short you are not educating the customer
- Problem: Experience a low win rate with long sales cycle
- Solution: You educate too much, and are talking clients “out of the deal”
- Problem: High win rate but with low deal flow
- Solution: Sales is too selective accepting leads. Educate salespeople on what to accept and how to overcome key obstacles.
STEP 8: Data-Driven Sales (must have enough data to be statistically significant)
STEP 9: Create your MRR Dashboards
LOGO MRR: Growth is primarily based on securing new revenue from newly acquired accounts, and renewing those accounts. This kind of growth chart is very common when you sell a platform of which the price is based on the number of seats. Although the seats may grow they often grow at a linear pace for most companies. The revenue churn is measured in 3-7%, but often related to Logo churn (2-3%).
USAGE MRR: Growth is primarily based on growing new revenue from existing customers – based on usage; Think of storage, templates, content, leads etc. In this case business can grow a lot faster based on the same amount of customers, but in turn it is more prone to churn as customers can turn-off/down usage overnight.
Both of the above dashboards should provide a quick idea of how the business is performing and both require a different “dashboard” approach.
STEP 10: What’s next? A closed loop system with a stronger focus on use/expansion revenue.
In the picture below you see the development of generations of data/dashboards as we obtain more data.
Generation 1 – Silos: The first generation of how data was used was in silos. Sales started first with just measuring deals and MRR. Marketing deepened it out in MQLs per campaign, and sales looked at deals won, average price etc.
Generation 2 – Connecting the silos: Today we are obtaining data across silo’s – such as how much pipeline did a marketing campaign generate? And which salesperson “closed” deals that churned? What was the sales cycle?
Generation 3 – Across silo’s: We are getting a first glimpse of data that shows causality and which helps us identify the impact across the business. Think of which marketing campaign generates the fastest business in June vs. August, or which sales rep has the shortest sales cycle but it results in high churn and low revenue expansion in the first years. We also see a trend shifting towards more detailed reporting on expansion revenue measurements and how it sourced vs. deal wins.
Generation 4 – Closing the loop: In an open loop system you are manually creating corrective action. As such, the data primarily feeds a dashboard which in turn is read by a person who has to make a decision. However, in closing the loop we can automate the process. Think of linking the results to Adwords, Facebook campaigns etc. As the results falter the system can suggest and/or take corrective action to impact short/long-term results.